Harnessing AI-Powered Business Intelligence for Business Analysis
Artificial Intelligence (AI) is transforming the landscape of business intelligence (BI), evolving it from a retrospective data tool into a proactive decision-making powerhouse. By integrating AI, organizations can shift from basic reporting to actionable intelligence, empowering business analysts to drive innovation, efficiency, and growth. This article delves deeply into how AI-powered BI tools reshape the decision-making process and propel organizations toward sustained success.
The Evolution of Business Intelligence and the AI Infusion
The journey of BI began in the 1970s with decision support systems designed for IT experts analyzing limited datasets. As the BI landscape matured, the mid-2000s ushered in self-service analytics, democratizing data access for non-technical users. However, the rapid expansion of data in volume, variety, and velocity has presented challenges that traditional BI systems cannot adequately address.
AI seamlessly bridges these gaps, enhancing BI with capabilities that automate tasks, analyze complex datasets and process unstructured data. These improvements empower business analysts to focus on high-value tasks such as strategy development and predictive modeling, which are critical for achieving organizational growth.
AI-Enhanced Descriptive and Diagnostic Analytics: Laying the Foundation
Descriptive analytics, traditionally at the heart of BI, involves understanding past data to identify trends and patterns. AI enhances this phase by automating labor-intensive tasks, such as anomaly detection and summarization of key metrics. Tools powered by natural language processing (NLP) enable business analysts to interact with data in a conversational manner, asking complex questions like, "How did last quarter’s sales compare across regions?" and receiving immediate, insightful responses.
Diagnostic analytics goes a step further by explaining why certain phenomena occurred. For example, decomposition trees and key influencer analysis allow analysts to uncover factors driving sales dips or customer churn. These capabilities help pinpoint root causes with minimal manual effort, enabling analysts to allocate their time to decision-making rather than data exploration.
Predictive Analytics: Transforming Forecasting into Strategic Advantage
Predictive analytics, driven by machine learning (ML), enables business analysts to anticipate future trends with unprecedented precision. Unlike traditional methods that rely on linear models, AI-powered predictive tools utilize complex algorithms capable of analyzing high-dimensional datasets. For instance, an AI system can analyze historical sales data, customer behavior, and external market conditions to predict revenue trends or churn probabilities with remarkable accuracy.
An advanced feature of AI-driven predictive analytics is its scalability. While traditional models might predict overall sales for the next quarter, AI allows granular forecasting—estimating individual customer behavior. This granularity is a game-changer for targeted marketing campaigns, allowing businesses to tailor interventions to retain high-value customers or upsell products to those likely to buy.
Prescriptive Analytics: Action-Oriented Recommendations
Prescriptive analytics represents the pinnacle of AI-powered BI, providing actionable recommendations based on predictive insights. By analyzing historical data and customer preferences, AI can suggest tailored actions to maximize outcomes. For example, it might recommend offering a discounted subscription plan to a customer flagged as having a high churn risk or proposing inventory adjustments based on demand forecasting.
Unlike traditional decision-making approaches, which often rely on intuition or static rules, prescriptive analytics combines real-time data analysis with historical patterns to deliver dynamic, context-sensitive recommendations. This enables organizations to respond swiftly to changes in the market, enhancing agility and competitiveness.
Unlocking the Value of Unstructured Data
AI-powered BI excels in processing unstructured data—a domain traditionally inaccessible to conventional BI systems. With advancements in computer vision and NLP, organizations can analyze customer reviews, social media posts, PDF documents, and even images or audio files.
This capability is invaluable for customer sentiment analysis. For example, an AI model can analyze thousands of customer reviews to identify common pain points and trends, providing actionable insights for product development or service improvement. Similarly, AI-driven image recognition tools can be employed in industries like retail and manufacturing to analyze product quality or monitor inventory levels in real time.
Democratizing Insights Across Organizational Hierarchies
One of the most profound impacts of AI-powered BI is the democratization of data insights. By integrating low-code or no-code interfaces, AI tools enable non-technical stakeholders, from marketing managers to C-suite executives, to interact with and interpret data without relying on data specialists. This democratization not only fosters a culture of data-driven decision-making but also accelerates the speed at which insights are acted upon across all organizational levels.
Moreover, AI-driven automation significantly reduces the workload for business analysts by handling repetitive tasks, such as data cleansing and report generation. This enables analysts to focus on strategic initiatives, ensuring that their expertise is leveraged to its fullest potential.
Augmenting Human Decision-Making: The AI-BI Synergy
AI-powered BI is not about replacing human decision-makers but augmenting their capabilities. The synergy between AI and human expertise creates a framework for informed and strategic decisions. For instance, while AI can predict a spike in customer churn rates, the insights need to be interpreted within the broader context of market dynamics and organizational goals—a task best suited for experienced business analysts.
Additionally, AI systems can identify opportunities that humans might overlook. By analyzing data at a granular level, these systems uncover patterns and correlations that might not be immediately apparent, providing analysts with a richer set of insights to work with.
Challenges and Ethical Considerations
Despite its advantages, implementing AI-powered BI is not without challenges. Data quality and governance remain significant hurdles. AI models are only as good as the data they are trained on; hence, organizations must invest in robust data management practices to ensure accuracy and reliability.
Ethical considerations are equally important. As AI systems influence decisions that affect employees, customers, and stakeholders, transparency and accountability must be prioritized. Business analysts play a crucial role in scrutinizing AI-generated insights to ensure they align with ethical standards and organizational values.
Best Practices for Implementing AI-Powered BI
To fully harness the potential of AI-powered BI, organizations should adopt the following best practices:
- Identify Strategic Use Cases: Prioritize areas where AI can have the most significant impact, such as customer retention, supply chain optimization, or market expansion strategies.
- Leverage Scalable Platforms: Invest in scalable AI-as-a-Service (AIaaS) and Automated Machine Learning (AutoML) platforms to expedite deployment and reduce costs.
- Cultivate Collaboration: Foster collaboration between data scientists, business analysts, and IT teams to ensure seamless integration of AI capabilities into BI workflows.
- Continuous Learning and Feedback: Establish feedback loops to monitor AI model performance and make iterative improvements over time.
- Focus on Change Management: Address resistance to AI adoption by providing training and demonstrating its value through pilot projects.
Conclusion
AI-powered BI represents a paradigm shift in how organizations approach decision-making. Automating routine tasks, uncovering hidden insights, and providing actionable recommendations enable business analysts to become strategic drivers of organizational growth. As AI technologies continue to advance, their integration with BI will unlock new possibilities, fostering innovation and competitiveness in an increasingly data-driven world.
You May Also Like
These Related Stories
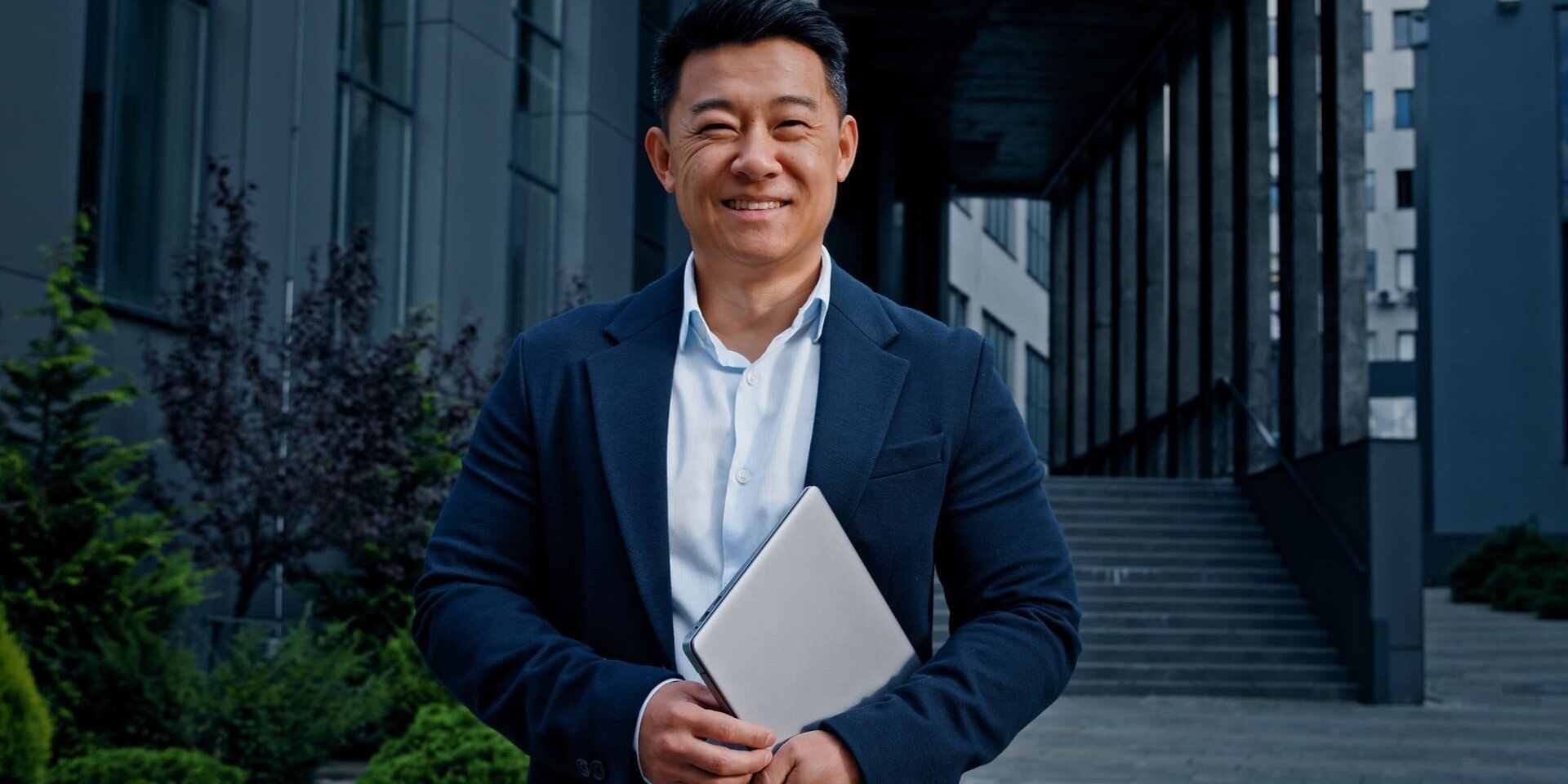
Can Business Analysts become Business Consultants?
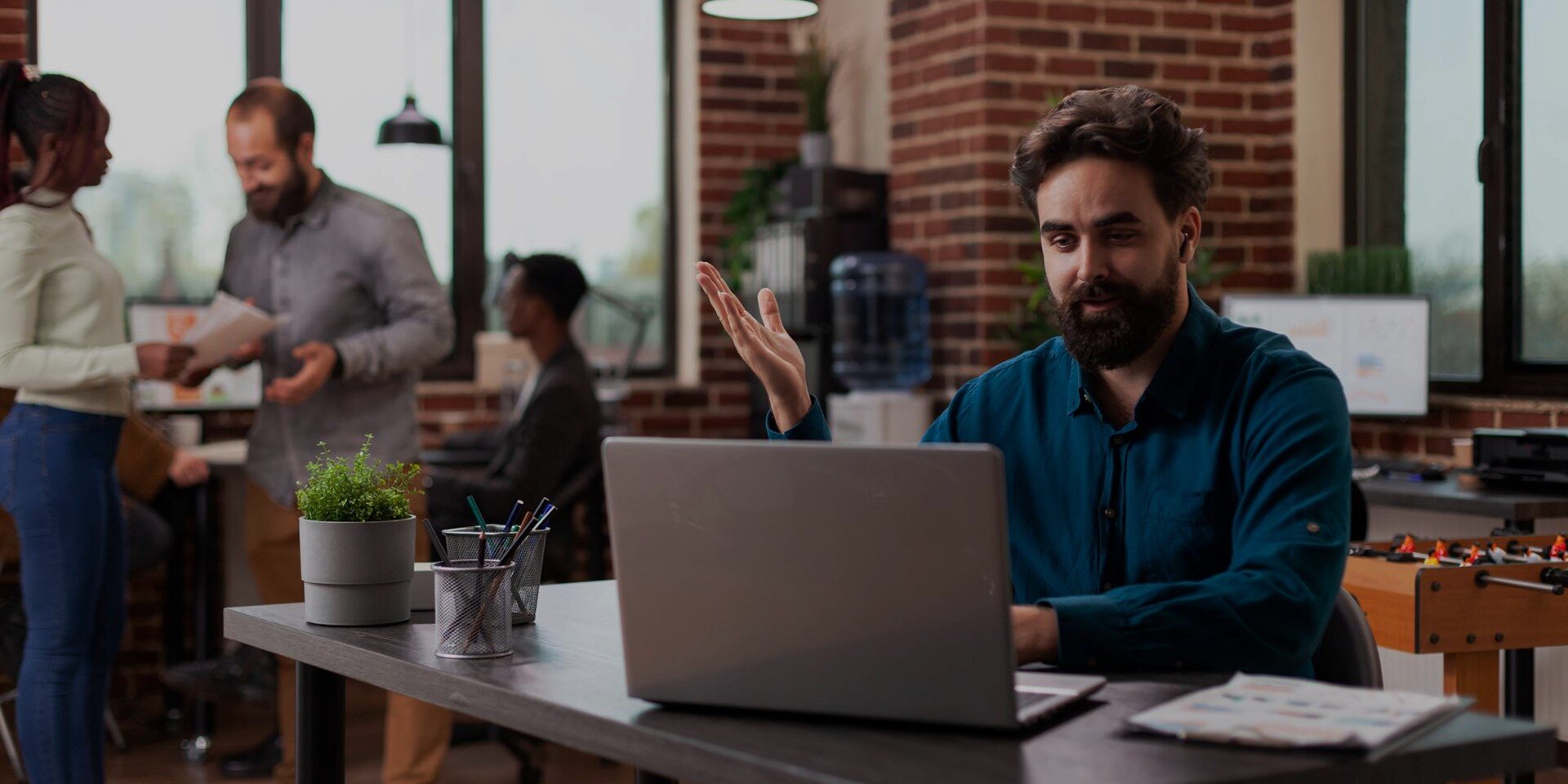
Choosing Business Analysis Approach Without Losing Your Hair
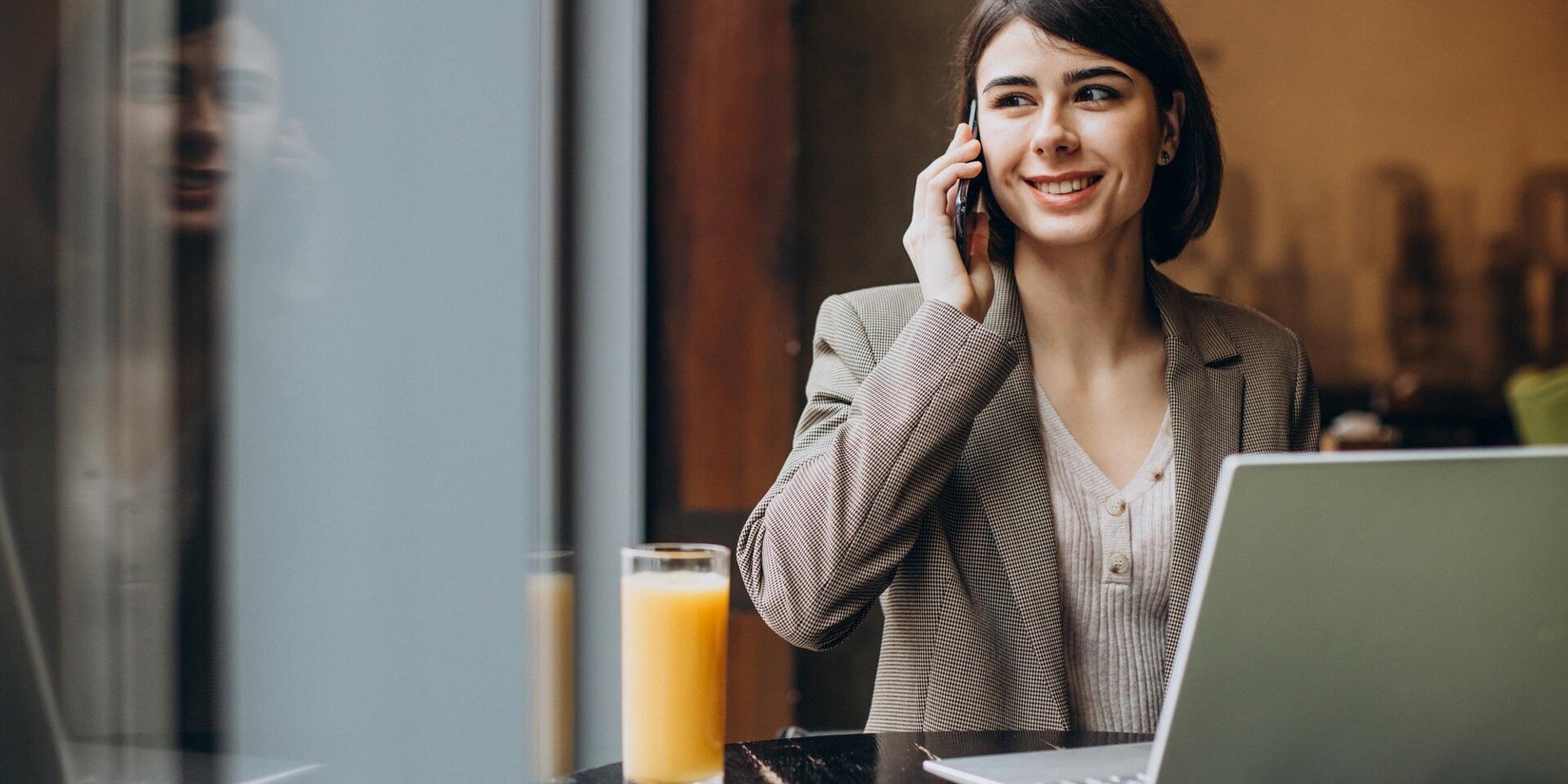
No Comments Yet
Let us know what you think